- 1. Complexity and Unpredictability of Financial Markets
- 2. Data Quality Challenges
- 3. Ethical Risks and Regulatory Challenges in AI-Driven Financial Trading
- 4. Security Risks and Cyber Threats to AI in Financial Trading
- 5. Technical Limitations of AI Algorithms in Financial Trading
- 6. Socioeconomic Impacts
- 7. Cost and Skill Barriers to AI Adoption in Financial Trading
- 8. Over-Reliance
- 9. Algorithmic Bias and Lack of Transparency in AI-Driven Financial Trading
- 10. Systemic Risks and Converging Algorithmic Behaviors in AI Trading
- Conclusion
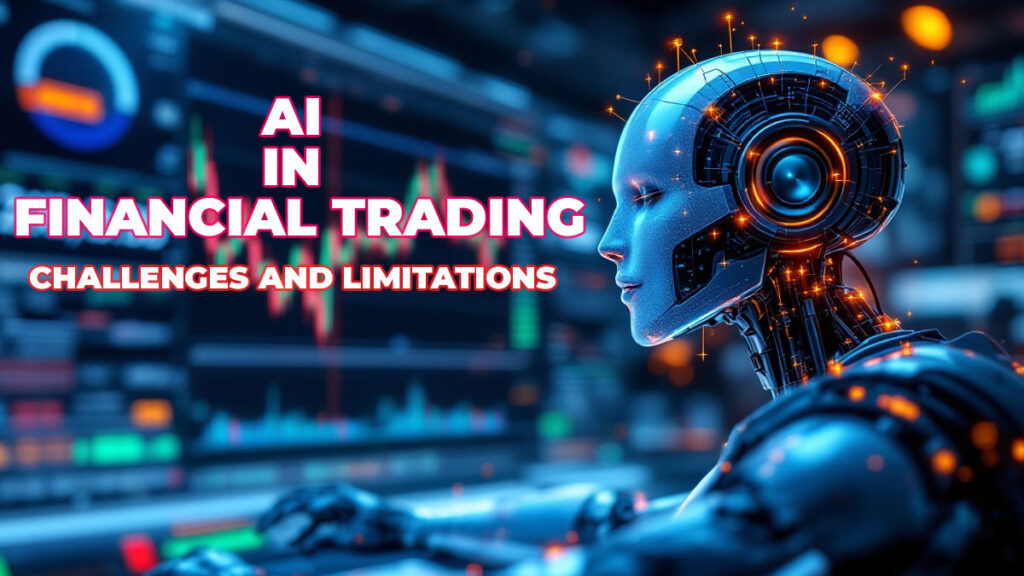
Artificial Intelligence (AI) has become one of the most transformative technologies across various industries, including financial trading. With advanced algorithms and machine learning techniques, AI in financial trading can analyze vast datasets and provide precise predictions about market trends and customer behaviors. However, despite its potential, AI in financial trading faces numerous challenges and limitations that could hinder its effectiveness. In this article, we explore these critical obstacles in detail.
1. Complexity and Unpredictability of Financial Markets
Financial markets are inherently complex and dynamic, influenced by multiple factors that can change unpredictably. Economic policies, global news, interest rates, and investor behavior can all significantly impact market trends in the short term.
Even the most advanced AI systems struggle to predict such sudden and unpredictable changes. AI in financial trading relies on historical data for training, but events like economic crises, natural disasters, or unexpected policy shifts can render historical patterns obsolete. This limitation means that Artificial Intelligence in financial trading may produce inaccurate predictions or fail to react appropriately to market disruptions.
2. Data Quality Challenges
The accuracy of AI in financial trading depends on the quality of its input data. Inconsistent, incomplete, or noisy data can lead to significant errors in analysis and decision-making.
Financial market data is often collected from diverse sources, creating challenges in ensuring consistency and integration. Furthermore, changes in data collection methods or formats require frequent algorithm updates and retraining. These issues make maintaining high-quality data a critical challenge for AI in financial trading.
3. Ethical Risks and Regulatory Challenges in AI-Driven Financial Trading
The implementation of AI in financial trading raises ethical concerns. AI’s automated decision-making can inadvertently promote speculative behavior, destabilize markets, or prioritize profits over broader economic stability.
Moreover, regulatory bodies have yet to establish comprehensive guidelines for the use of Artificial Intelligence in financial trading. This lack of clarity creates uncertainty for companies, which may face penalties or compliance issues. Addressing these ethical and regulatory concerns is essential for the responsible use.
4. Security Risks and Cyber Threats to AI in Financial Trading
Artificial Intelligence systems in financial trading are vulnerable to cyberattacks, posing a significant risk to institutions and users. Hackers could manipulate algorithms to serve their interests or tamper with input data to distort predictions.
As AI becomes a primary tool in financial decision-making, safeguarding algorithms and data from cyber threats becomes increasingly critical. A breach could lead to substantial financial losses and market instability, emphasizing the need for robust security measures in Artificial Intelligence-driven financial trading systems.
5. Technical Limitations of AI Algorithms in Financial Trading
Artificial Intelligence in financial trading faces several technical challenges. Traditional models often struggle to recognize complex patterns and nonlinear relationships in financial data. For instance, simple regression models or basic neural networks may fail to capture the intricate and dynamic behavior of financial markets.
Advanced models, such as deep learning and generative networks, offer better performance but require significant computational resources and extensive datasets for training. These technical limitations hinder the widespread adoption of AI in financial trading, particularly for smaller firms.
6. Socioeconomic Impacts
The widespread use of AI in financial trading could lead to significant socioeconomic consequences. Automation might eliminate many jobs in the financial sector, increasing unemployment rates and raising concerns about workforce displacement.
Additionally, if a few advanced algorithms dominate financial markets, it could result in market concentration and reduced competition. This monopolistic behavior could destabilize economies and erode trust in financial systems.
7. Cost and Skill Barriers to AI Adoption in Financial Trading
Successfully implementing Artificial Intelligence in financial trading requires a combination of technical expertise and financial resources. The shortage of professionals skilled in AI, finance, and ethical compliance adds to the challenge.
Moreover, the high costs associated with AI adoption—such as acquiring powerful hardware, storing large datasets, and updating algorithms—make it difficult for smaller firms to compete. These barriers slow down the broader adoption.
8. Over-Reliance
Relying too heavily on AI in financial trading can lead to a decline in human creativity and decision-making skills. When decisions are based solely on AI outputs, human analysts may lose their ability to think critically and adapt to unexpected situations.
This over-reliance can become a liability during crises, as humans might struggle to step in and address issues that AI systems fail to resolve.
9. Algorithmic Bias and Lack of Transparency in AI-Driven Financial Trading
AI in financial trading is prone to algorithmic biases. If the training data contains historical biases, the algorithms may replicate and reinforce these patterns, leading to unfair or discriminatory decisions.
Furthermore, many AI systems, particularly those using deep learning or reinforcement learning, operate as “black boxes.” Their decision-making processes are often opaque, making it difficult for users to understand or trust their outputs. This lack of transparency can undermine confidence in AI-driven financial trading.
10. Systemic Risks and Converging Algorithmic Behaviors in AI Trading
This can introduce systemic risks when algorithms behave similarly during market events. For instance, if many firms use similar AI models, their trading behavior may converge, leading to simultaneous buying or selling.
This phenomenon, known as “algorithmic flash crashes,” can cause extreme market volatility and significant financial losses. To mitigate such risks, developers must design AI systems that promote diverse decision-making strategies, especially during emergencies.
Conclusion
AI in financial trading offers numerous advantages, including enhanced accuracy, faster processing, and better market trend predictions. However, these benefits come with significant challenges, from data quality issues to ethical concerns and systemic risks.
To fully harness the potential , companies and regulators must work together to address these challenges. By developing robust security measures, ethical frameworks, and diverse algorithms, the financial sector can ensure that AI contributes to a more stable and efficient trading environment.
Related Topic :